Hey there, tech-savvy friend! If you’ve ever stumbled upon the term "AAGMALS" and wondered what it means, you’ve landed in the right place. AAGMALS is more than just an acronym—it’s a game-changer in the world of advanced analytics and machine learning systems. Whether you’re a tech enthusiast, a developer, or someone curious about the future of artificial intelligence, this article will break it down for you in a way that’s easy to digest yet packed with valuable insights.
Now, let’s get real. AAGMALS isn’t just another buzzword in the tech industry. It’s a framework that’s gaining traction because of its ability to streamline complex processes and deliver actionable insights. Think of it as a Swiss Army knife for data scientists and analysts who need to tackle big data challenges without breaking a sweat. In this guide, we’ll dive deep into what AAGMALS is, why it matters, and how you can leverage it for your projects.
But hold up—before we jump into the nitty-gritty, let me assure you that this isn’t going to be one of those overly technical articles that leaves you scratching your head. We’ll keep it conversational, practical, and filled with real-world examples. So, grab a cup of coffee, and let’s unravel the mystery behind AAGMALS together!
Read also:Morgan Vera Onlyfans Leaks The Truth Behind The Headlines
Here’s a quick roadmap of what we’ll cover:
- What is AAGMALS?
- The History and Evolution of AAGMALS
- Key Components of AAGMALS
- Benefits of Using AAGMALS
- How to Implement AAGMALS
- Common Challenges and Solutions
- Real-World Use Cases
- Future Trends in AAGMALS
- Tools and Technologies for AAGMALS
- Wrapping It All Up
What is AAGMALS?
Alright, let’s kick things off by defining what AAGMALS really is. Simply put, AAGMALS stands for "Advanced Analytics and Generative Machine Learning Algorithms System." It’s a framework designed to help organizations harness the power of data analytics and machine learning in a more efficient and scalable way. The beauty of AAGMALS lies in its ability to adapt to various industries, from healthcare to finance, retail, and beyond.
Here’s the deal: traditional data analytics systems often fall short when it comes to handling massive datasets or generating predictive insights. AAGMALS bridges that gap by incorporating cutting-edge technologies like generative AI and deep learning algorithms. This means you can not only analyze data but also create new, meaningful outputs based on patterns and trends.
But why should you care? Well, imagine being able to forecast market trends, personalize customer experiences, or even predict equipment failures before they happen—all with minimal effort. That’s the magic of AAGMALS in action.
The History and Evolution of AAGMALS
Early Beginnings
Believe it or not, the concept of AAGMALS didn’t just pop up overnight. Its roots trace back to the early days of machine learning and data analytics. Back in the late 2000s, researchers were already experimenting with ways to combine statistical models with machine learning techniques. However, these early systems lacked the scalability and flexibility that modern businesses demand.
The Rise of Generative Models
Fast forward to the 2010s, and we saw a major breakthrough with the advent of generative models like GANs (Generative Adversarial Networks). These models allowed machines to generate realistic outputs, such as images, text, and even music. This was a game-changer for industries that relied heavily on content creation and innovation.
Read also:Lyracr0w0 Onlyfans Leaks The Untold Story Behind The Viral Sensation
By the mid-2020s, the need for an integrated system that combined analytics with generative capabilities became apparent. And that’s how AAGMALS was born—a fusion of advanced analytics and generative machine learning algorithms designed to meet the demands of today’s data-driven world.
Key Components of AAGMALS
Now that we’ve covered the basics, let’s break down the key components of AAGMALS. Think of it as a well-oiled machine made up of several critical parts:
- Data Ingestion: This is where raw data from various sources is collected and processed for analysis.
- Preprocessing: Data cleaning and transformation to ensure accuracy and consistency.
- Analytics Engine: The brain of the system, responsible for performing complex calculations and generating insights.
- Generative Models: Algorithms that create new outputs based on learned patterns.
- Deployment: The process of integrating AAGMALS into existing systems for real-world applications.
Each of these components plays a vital role in making AAGMALS work seamlessly. Without them, the system wouldn’t be able to deliver the results it promises.
Benefits of Using AAGMALS
So, why should you consider adopting AAGMALS for your organization? Let’s take a look at some of the top benefits:
- Improved Efficiency: AAGMALS automates repetitive tasks, freeing up your team to focus on more strategic initiatives.
- Enhanced Decision-Making: With accurate insights at your fingertips, you can make smarter, data-driven decisions.
- Cost Savings: By optimizing processes and reducing errors, AAGMALS can help cut costs significantly.
- Competitive Advantage: Stay ahead of the competition by leveraging the latest advancements in AI and machine learning.
These benefits aren’t just theoretical—they’ve been proven time and again in real-world scenarios. Companies that have implemented AAGMALS have reported impressive results, from increased revenue to improved customer satisfaction.
How to Implement AAGMALS
Step 1: Assess Your Needs
Before diving headfirst into AAGMALS, it’s crucial to assess your organization’s specific needs. What problems are you trying to solve? What goals do you want to achieve? Answering these questions will help you tailor the implementation process to your unique situation.
Step 2: Choose the Right Tools
There’s no one-size-fits-all solution when it comes to AAGMALS. Depending on your requirements, you may need to select specific tools and technologies that align with your objectives. Some popular options include TensorFlow, PyTorch, and Scikit-learn.
Step 3: Train Your Team
Implementing AAGMALS isn’t just about technology—it’s also about people. Make sure your team is well-trained and equipped to handle the new system. This might involve workshops, online courses, or even hiring experts to guide the transition.
Common Challenges and Solutions
Of course, no technology is without its challenges. Here are some common hurdles you might face when implementing AAGMALS and how to overcome them:
- Data Quality Issues: Poor-quality data can lead to inaccurate insights. Solution? Invest in robust data cleaning and preprocessing techniques.
- Integration Complexity: Integrating AAGMALS with existing systems can be tricky. Consider working with experienced consultants to ensure a smooth transition.
- Resource Constraints: Limited budgets or personnel can slow down implementation. Prioritize your needs and focus on high-impact areas first.
Remember, challenges are opportunities in disguise. By addressing these issues proactively, you’ll set yourself up for long-term success.
Real-World Use Cases
To give you a better idea of how AAGMALS works in practice, here are a few real-world use cases:
- Healthcare: Predicting patient outcomes and personalizing treatment plans.
- Finance: Detecting fraud and optimizing investment strategies.
- Retail: Enhancing customer experiences through personalized recommendations.
These examples illustrate the versatility and potential of AAGMALS across different industries.
Future Trends in AAGMALS
As technology continues to evolve, so does AAGMALS. Here are some trends to watch out for:
- Edge Computing: Processing data closer to the source for faster insights.
- Explainable AI: Making AI models more transparent and understandable.
- Quantum Computing: Unlocking new possibilities for complex computations.
Exciting times lie ahead for AAGMALS enthusiasts!
Tools and Technologies for AAGMALS
When it comes to AAGMALS, having the right tools is essential. Here are some of the top tools and technologies you should consider:
- TensorFlow: A powerful open-source platform for machine learning.
- PyTorch: Known for its flexibility and ease of use.
- Scikit-learn: Great for traditional machine learning tasks.
Each tool has its strengths, so choose wisely based on your specific needs.
Wrapping It All Up
And there you have it—a comprehensive guide to understanding and implementing AAGMALS. From its definition and history to its benefits and challenges, we’ve covered everything you need to know to get started. Remember, AAGMALS isn’t just a tool—it’s a mindset that empowers organizations to unlock the full potential of their data.
So, what’s next? If you’ve found this article helpful, don’t forget to share it with your network. And if you have any questions or feedback, drop a comment below—we’d love to hear from you! Stay tuned for more insights into the world of AI and machine learning. Until next time, keep exploring and innovating!
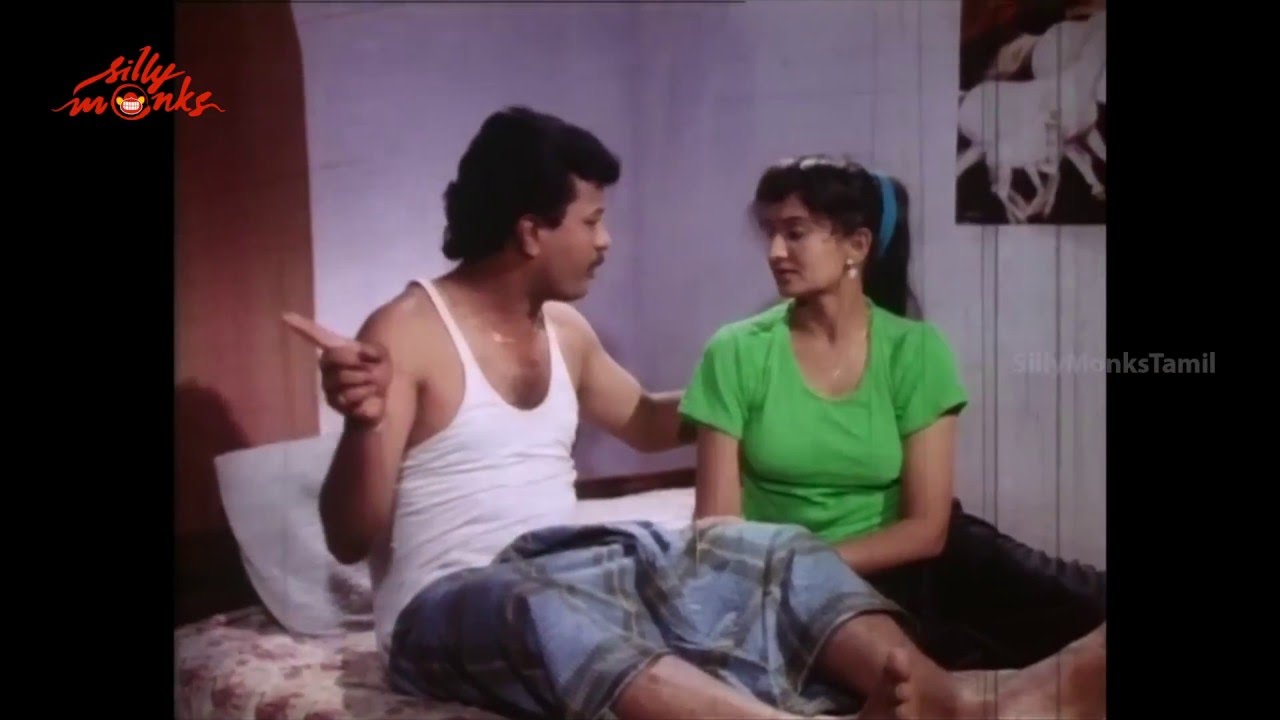